Blog
Data for growth
Analytics and data management for high-growth SMBs
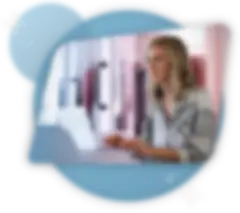
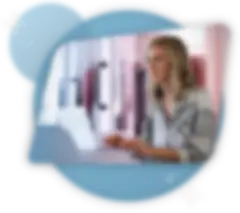
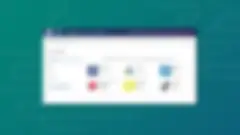
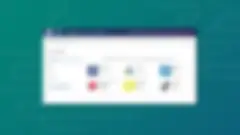
Winter '23 — what's new for Stitch?
By Thibaut Gourdel
Explore what’s new in the Stitch Winter ’23 release, including a connector to GA4 and beta connectors for TikTok and Snapchat ads. There’s also innovation around Snowflake, plus access management and pipeline monitoring enhancements.
Read more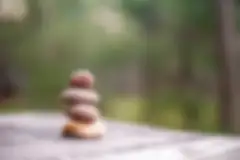
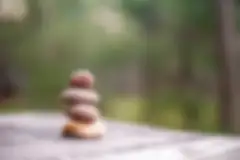
Balancing data sharing and compliance is hard - but it doesn't have to be
By Kensuke Ishii
Achieving data excellence doesn't have to be hard - here's how to balance sharing data while keeping compliant.
Read more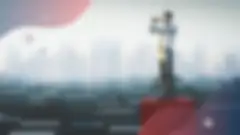
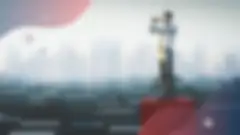
Embrace the future — make the move from descriptive to prescriptive analytics
By Jelena Tarbuk
The future is prescriptive - but how do you get there?
Read more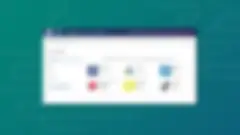
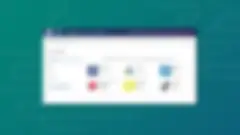
Winter '23 — what's new for Stitch?
By Thibaut Gourdel
Explore what’s new in the Stitch Winter ’23 release, including a connector to GA4 and beta connectors for TikTok and Snapchat ads. There’s also innovation around Snowflake, plus access management and pipeline monitoring enhancements.
Read more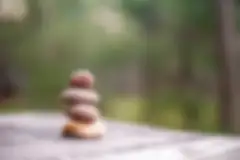
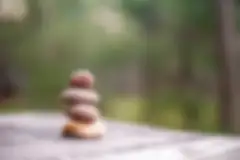
Balancing data sharing and compliance is hard - but it doesn't have to be
By Kensuke Ishii
Achieving data excellence doesn't have to be hard - here's how to balance sharing data while keeping compliant.
Read more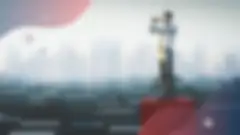
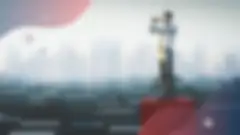
Embrace the future — make the move from descriptive to prescriptive analytics
By Jelena Tarbuk
The future is prescriptive - but how do you get there?
Read more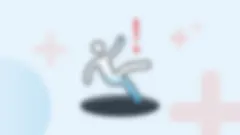
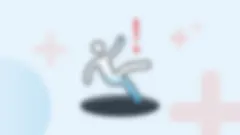
4 pitfalls in your data strategy (and how to avoid them)
By Talend Team
There’s no universal data strategy for all organizations — wouldn’t that be great? — but Talend has identified common points of failure that all organizations can watch out for. If you don’t avoid these pitfalls, repairing the damage could be costly.
Read more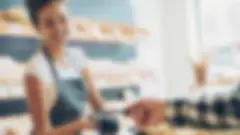
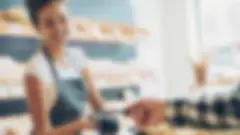
Retailers must leverage data to survive this pandemic
By Jamie Kiser
Jamie Kiser, COO and CCO at Talend, explains why retailers, striving not to miss out on future opportunities, must leverage one thing: data.
Read more